Buckling up for the long ride: embedding foundational AI into the future of transportation. A contribution by Jonas Boehm, TWAICE
Below, a contribution by Jonas Boehm, Partnerships Manager at battery analytics company TWAICE. AI and IoT are on course to revolutionize the transportation industry. Only those preparing to integrate these technologies can hope to come out as winners. Important applications can already be seen today through autonomous driving, crowd management or predictive maintenance. Although these […]
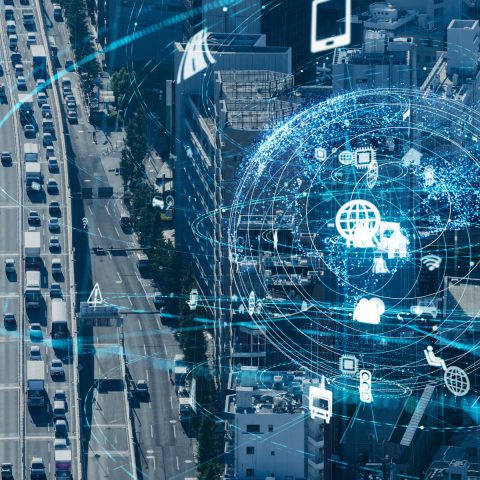
Below, a contribution by
Jonas Boehm, Partnerships Manager
at battery analytics company TWAICE.
AI and IoT are on course to revolutionize the transportation industry. Only those preparing to integrate these technologies can hope to come out as winners. Important applications can already be seen today through autonomous driving, crowd management or predictive maintenance. Although these tools are in every operator’s conversation, AI and IoT have yet to become an everyday reality for many incumbents.
As Mark Weiser famously said: “The most profound technologies are those that disappear. They weave themselves into the fabric of everyday life until they are indistinguishable from it”. The industry is still far from this stage of seamless integration as the long-term challenges surface: how to embed AI in their organization to create foundational value, and what will the new reality look like?
This article delves into four implications of AI for operators and bus owners and how they can buckle up for the long ride.
The transportation industry is a prime example of a transformative area and a perfect storm where autonomy, connectivity and electrification converge. The use of AI will be especially prevalent in areas where these new technologies converge. For example, AI optimization will be especially relevant to manage scaling electric fleets from diverse manufacturers, complex charging operations and to monitor electric assets
1 – AI will be business critical in transformative areas
The transportation industry is a prime example of a transformative area and a perfect storm where autonomy, connectivity and electrification converge. In other words, transformation is a daily occurrence where many things change simultaneously, introducing unprecedented complexity. At the same time, the changes bring about vast new possibilities of managing the system through data.
Hence, the use of AI will be especially prevalent in areas where these new technologies converge. For example, AI optimization will be especially relevant to manage scaling electric fleets from diverse manufacturers, complex charging operations and to monitor electric assets. Optimal bus operation is already commonly determined through various machine learning techniques, such as the number of buses and their schedules. AI will especially affect these business areas where changes are common.
2 – AI readiness over AI capability
In anticipation of such profound technological changes, creating AI readiness will be key. Businesses trying to develop their own AI capabilities in-house will find themselves unable to achieve comparable benefits as with the scalable hyper-specific solutions that are increasingly available.
Battery health is such an overlooked foundational topic and a black box for many in the industry. A degradation graph estimating the life of a bus battery can appear innocuous, but it is the result of AI processing 2.4 billion data points over the lifetime of a bus in a complex system where operators, manufacturers, telematics providers and AI-providers work in sync.
Instead of focusing on own AI capability, enabling AI readiness will be the hinge of effective integration. The shift from capability to readiness requires a shift in mindset for these teams and their management: they must be empowered to share data with AI providers, understand where their comparative advantage lies – for example in their specific domain knowledge, and be able to build on and integrate analytics from external providers.
3 – AI and IoT are a business challenge
AI and IoT will not be a technical challenge but a business one. Technical challenges are already being addressed today, for example through the easy access of potent cloud services. However, the real difficulty will be taking AI from the tech space into cross-functional and cross-organizational working groups. This means connecting with manufacturers, asset owners and telematics providers, which will only be possible if shared value can be created.
Sharing data and aligning on data integration methods will be required; think of Nick Clegg’s statement that data, unlike oil – a finite commodity – does not have value when hoarded. Different datasets will have to be integrated and applicable business cases drawn. Finally, trust will have to be established through AI and IoT such that these partnerships are future-proof. In essence, these problems will be business-related. This is not a trivial task and is often the real road blocker to introducing profound AI-cases into operations.
4 – AI will be foundational
Finally, winning AI and IoT technologies will focus on foundations. While AI can optimize operational issues efficiently, some of the most important cases of AI will solve profoundly fundamental issues while enabling more use cases on top. Battery health, for example, is such an overlooked foundational topic and a black box for many in the industry. A degradation graph estimating the life of a bus battery can appear innocuous, but it is the result of AI processing 2.4 billion data points over the lifetime of a bus in a complex system where operators, manufacturers, telematics providers and AI-providers work in sync.
The information it provides, however, is far more telling and can contribute to essential conversations such as ideal battery swapping, better investment decisions, and warranty planning. Moreover, foundational subjects can be built upon. Improved scheduling can be introduced to maximize battery lifetime. Driver behavior can be monitored and improved. The list of possibilities goes on. Battery analytics is a foundational AI use case.
AI and IoT will not be a technical challenge but a business one. Technical challenges are already being addressed today, for example through the easy access of potent cloud services. However, the real difficulty will be taking AI from the tech space into cross-functional and cross-organizational working groups. This means connecting with manufacturers, asset owners and telematics providers, which will only be possible if shared value can be created. Sharing data and aligning on data integration methods will be required.
AI and IoT are words in everyone’s mouths in the transportation industry now and will be for years to come. However, they have yet to be meaningfully embedded in operations. Incumbents need to recognize their importance and reflect on Weiser’s words to “weave” them into their organization. By enabling battery analytics capabilities and other foundational AI use cases, operators will buckle in for AI and IoT’s long ride.