Artificial intelligence as a mean to optimize vehicles’ charging. An interview with BluWave-ai
How can the use of AI generate cost reductions and improve battery lifespan? In the transition from ICE vehicles to electric ones, operators face several challenges. New parameters come into play. And Artificial Intelligence could play a major role. We discussed the topic with Devashish Paul, CEO of BluWave-ai. The company, headquartered in Canada and active […]
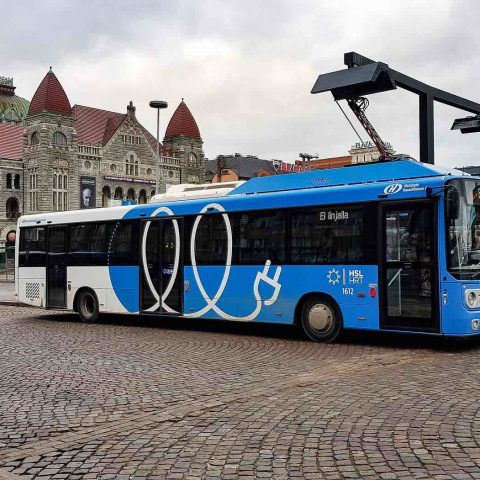
How can the use of AI generate cost reductions and improve battery lifespan? In the transition from ICE vehicles to electric ones, operators face several challenges. New parameters come into play. And Artificial Intelligence could play a major role.
We discussed the topic with Devashish Paul, CEO of BluWave-ai. The company, headquartered in Canada and active in the field of grid energy optimization platforms, was established in 2017 «around the idea of bringing innovations in artificial Intelligence, edge computing, and cloud-based super-computing to the world of distributed renewable energy», as the company itself put it. And BluWave-ai, in a recent paper, esemplifies the change underway in the mobility sector as follows: «The electric utility becomes the gas station».
BluWave: the electric utility becomes the gas station
Mr. Devashish Paul, how using artificial intelligence can boost wide-spread fleet electrification?
«The challenges of running a fleet of vehicles is something fleet operators are very familiar with, but when the electric utility becomes their gas station it introduces a whole new level of complexity. The usual service and operational schedules now have to be integrated with new parameters such as contractual and time-of-use electricity price, peak demand constraints, in-house versus on-route charging, weather and traffic conditions, to name a few. Optimizing energy use across these multi-variable and multi-agent problems with continuously changing environments is an ideal job for a data-driven solution enabled by artificial intelligence (AI).
By adopting an AI-enabled energy optimization system, fleet operators can reduce both capital expenses (by right-sizing the amount of energy sources and energy storage facilities they require upfront) and operating expenses (by adapting charging times to the most favorable energy pricing). It also enables them to add renewable power sources to the mix, and plan according to a reliable prediction of their energy generation».
To what extent could the use of AI could impact on TCO (total cost of ownership) models of e-buses?
«It could bring to reductions in capital expenses (CAPEX), thanks to the reduction in the facility upgrades needed to add extra energy supply and to the reduction in battery costs, due to AI optimization to maximize battery lifespan in vehicles. Secondly, we should mention a cut in operating expenses (OPEX), for instance thanks to a reductions in net energy costs via holistic charging strategies. Also, additional operating improvements can be achieved via Intelligent management of electric fleet in order to maximize fleet uptime, revenue generating kilometers, and maximizing utilization of charging infrastructure. Finally, optimizing the battery lifetime through smart charging cycles».
In Europe we are witnessing a trend towards depot charging technology, with buses equipped with large batteries and decreasing adoption of en-route charging technologies. How do you see such a development and which trends are you observing in other areas of the world?
«In the operators we’ve been talking to in North America we have mostly seen centralized charging at depots. In Dubai, the Roads and Transportation Authority (RTA) is testing dynamic wireless charging of electric vehicles and buses in motion using the Shaped Magnetic Field in Resonance (SMFIR) technology. This allows the buses to charge while parked on the road and in motion.
The requirement for the en-route charging varies from one demographic to another. Whether it is a suitable option or not depends on various factors like size of the batteries, the length of the routes, traffic conditions, and operating constraints of the fleet. For example, one may decide to charge a vehicle multiple times in shorter durations aligning with times of lower demand rather than a longer duration and fully charging the batteries.
Also, en-route charging could contribute to prolonging the battery lifetime by keeping the depth of discharging at higher levels (since the lower the depth of discharge, the higher the battery degradation). However, this brings additional complexity to the fleet manager making charging decisions more complex».
Artificial intelligence and electric bus deployment
The adoption of e-buses in public transport (and, eventually, the related AI-based technology) is based on tender requirements. Do you see PT authorities mindset being ready for such a challenge?
«Although the mindset of the public transit authorities is by no means our expertise, we do see a desire for modernizing fleets and engaging pilot projects to test the waters. Likely we’ll see mixed vehicle fleets (diesel or traditional fuels plus electric) as the standard for the near future and here AI can also help with optimizing energy use. Of course, the pandemic has put these authorities and many others in a more cautious mode for new ventures. At the same time we’ve found there is still momentum. The pandemic shutdowns led to cleaner air and clearer skies in urban areas worldwide, reminding people of how much healthier the air they breathe can be. We’re hoping this will motivate industry and governments to increase incentives to reduce fossil fuel consumption. Vehicle electrification and using green fuel sources can have a significant impact».
How can the use of AI influence battery lifespan?
«EV batteries represent a major capital cost, so prolonging battery life will yield significant savings and improve the return on investment (ROI) for the EVs. A rule-based (non-AI) energy management system may deliver short-term savings but result in reduced battery life and therefore higher costs over the battery life-time. Accounting for battery degradation with conventional optimization techniques results in non-linear formulations which are either too complex to solve or computationally infeasible. Alternatively, an AI-enabled energy management system can effectively incorporate the battery manufacturer’s operational constraints pertaining to the battery degradation, such as charge and discharge ramping rates and acceptable ranges of state-of-charge and operating temperature».
May you give a practical example of holistic approach that include AI-based intelligent energy management applied to public transport?
«We could mention the following examples:
- Allow transport operators to take advantage of intra-day electricity price fluctuations
- Fleet operators can optimize charging costs by taking advantage of their utility’s price fluctuations throughout the day.
- Optimization shifts EV charging time from high-price to low-price periods, bringing considerable savings to the fleet operator.
- This is achieved by an intelligent coordination of EVs’ charging time, based on their availability and state-of-charge, as well as implementation of a smart battery energy storage system (BESS) at the depot.
- Respond to grid operator’s price and demand response signals
- Fleet electrification will have a profound impact on electricity demand peaks, producing spike periods due to the simultaneous grid connection of large batteries. The current distribution infrastructure is not designed to handle such spikes, exposing it to unacceptable voltage profiles and significant outages.
- In an attempt to defer costly infrastructure upgrades, some grid operators have already introduced incentive-based customer programs, such as demand response ones, aimed at reducing or shifting the demand during peak periods.
- Being able to participate in demand response programs enables the fleet operator to negotiate more favorable pricing contracts upfront, as well as realizing considerable savings in supplied cost of energy.
- An AI-enabled predictive optimizer can increase the savings further through an accurate prediction of on-peak periods and mitigation of other systemic uncertainties.
- Add Renewable Energy to the Mix
- Distribution depots and bus terminal buildings can host roof-top solar PV, producing locally-generated energy. The PV supply can directly charge vehicles and/or be stored in a battery.
- Alternatively, a growing number of corporations and transit authorities enter into power purchasing agreements (PPAs) with independent power producers (IPPs), to source renewable energy at attractive rates.
- However, renewables resources are generally non-dispatchable and intermittent sources of energy, and as such the supply may not coincide with demand. This calls for the use of an intelligent predictive coordination mechanism to manage the charging and discharging of EVs and BESS as well as other controllable assets, aiming at a smooth net-demand profile and lower cost of supplied energy.
- Aggregate all loads at charging depot
- Fleet operators often control loads other than EVs, including infrastructure ones such as lighting and heating, ventilation, and air conditioning (HVAC) systems at bus terminals, distribution centers and warehouses.
- An AI-enabled aggregator can discover patterns otherwise undetectable to operators or conventional controllers, and make use of such patterns to arrive at more optimized dispatch decisions.
- Account for the effect of traffic on charging requirements and fleet routing
- Utilizing a variety of third party data sources, an AI optimizer may also recognize and act according to complex interactions between weather on traffic patterns, or intelligent utilization of en-route and depot-based charging assets based on historical and live traffic. If an AI model predicts that traffic may be busier than usual, then routes can be adjusted to ensure that batteries leave the depot with higher charge, or routes can be adjusted so that buses spend more time charging on opportunistic charging stations»